水利要闻
科学研究
工作动态
通知公告
科研平台
- 国家大坝安全工程技术研究中心
- 江湖保护与水安全保障国际科技合作基地
- 国家引才引智示范基地(社会与生态建设类)
- 水利部长江中下游河湖治理与防洪重点实验室
- 水利部岩土力学与工程重点实验室
- 水利部水工程安全与病害防治工程技术研究中心
- 水利部山洪地质灾害防治工程技术研究中心
- 水利部长江江源区水生态系统野外科学观测研究站
- 流域水资源与生态环境科学湖北省重点实验室
- 长江水利委员会长江经济带保护与发展战略研究中心
- 武汉市智慧流域工程技术研究中心
雷竞技raybet在线下载
水利与科技

学术团体
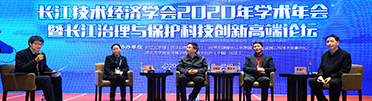
- 全球水伙伴(中国 长江)
- 长江技术经济学会
- 长江治理与保护科技创新联盟
- 中国岩石力学与工程学会岩石力学测试专业委员会
- 中国疏浚协会水库生态疏浚与资源化利用专业委员会
- 湖北省岩石力学与工程学会
- 湖北省水力发电工程学会水工水力学专业委员会
- 重庆岩石力学与工程学会岩石工程测试技术专业委员会